Written by Tilde Jaques, MDI Journalism Intern
The Massive Data Institute (MDI)’s 2023-2024 Scholars presented their projects at the annual spring research showcase on Wednesday, May 1.
The MDI Scholars Program, launched in 2019 as an experiential learning opportunity for undergraduate and Master’s students at Georgetown, supporting them in their work alongside researchers and practitioners in interdisciplinary fields to engage in data science and public policy research. This year, MDI partnered with Georgetown’s Ethics Lab for the scholars cohort to more intentionally integrate ethics into the policy and data science research projects. MDI Director Lisa Singh explains that this was an effort to “have our students consider the ethical implications of the questions they’re researching, the data they’re using, and the technologies they’re developing.”
In her opening remarks, Dean Maria Cancian of the McCourt School of Public Policy shared that “one of the things that is really hard and amazingly successful in this program is the ability to bring together extraordinary faculty, amazing agency partners and real practical problems, and students from across the university.”
The 2023-2024 cohort of 30 students worked on 17 different research projects ranging in topics from environmental justice to social media bias. At the research showcase, each team of scholars presented the research they conducted under their advisor with a poster and a 60-second flash talk explaining their findings.
As the 2023-2024 academic year comes to a close, the Summer 2024 Scholars will begin their interdisciplinary research projects at the end of the month.
Below is a summary of the MDI Scholar Research Posters; each poster title links to the full poster online.
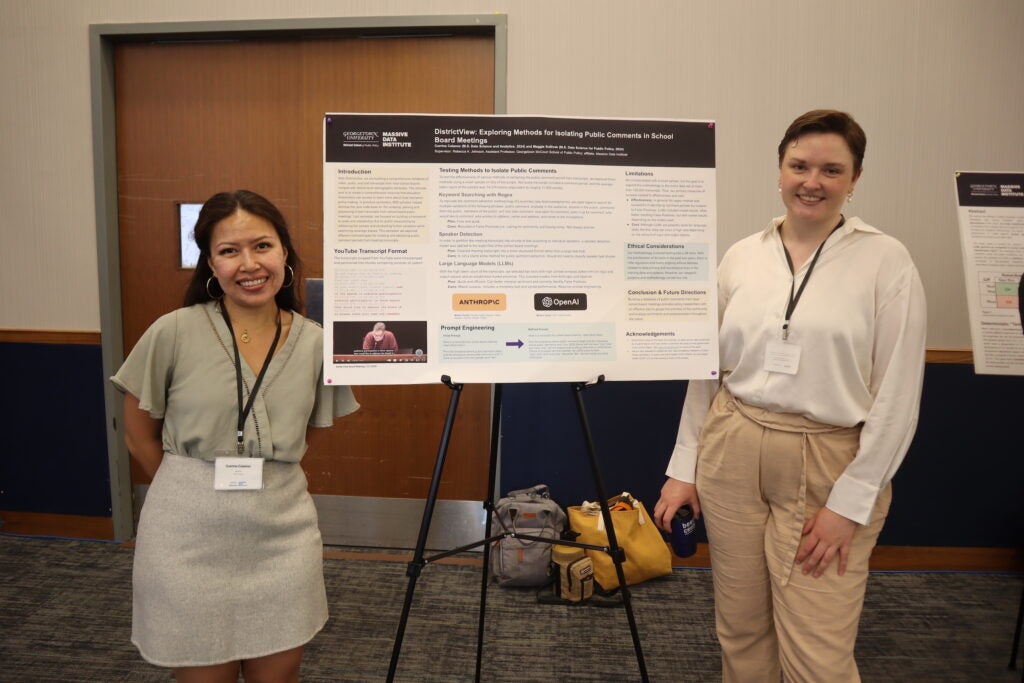
Corrina Calanoc (MS-DSAN ’24) and Maggie Sullivan (MS-DSPP ’24): DistrictView: Exploring Methods for Isolating Public Comments in School Board Meetings.
Calanoc and Sullivan continued their work with Professor Rebecca Johnson on a project that aimed to create a database of information from school board meetings to make a comprehensive resource for policymakers to learn more about local education. Calanoc explains that because “a lot of what we know about local education policy from the news is about very polarizing topics,” this semester they focused on identifying the polarizing topic areas from public comments during school board meetings.
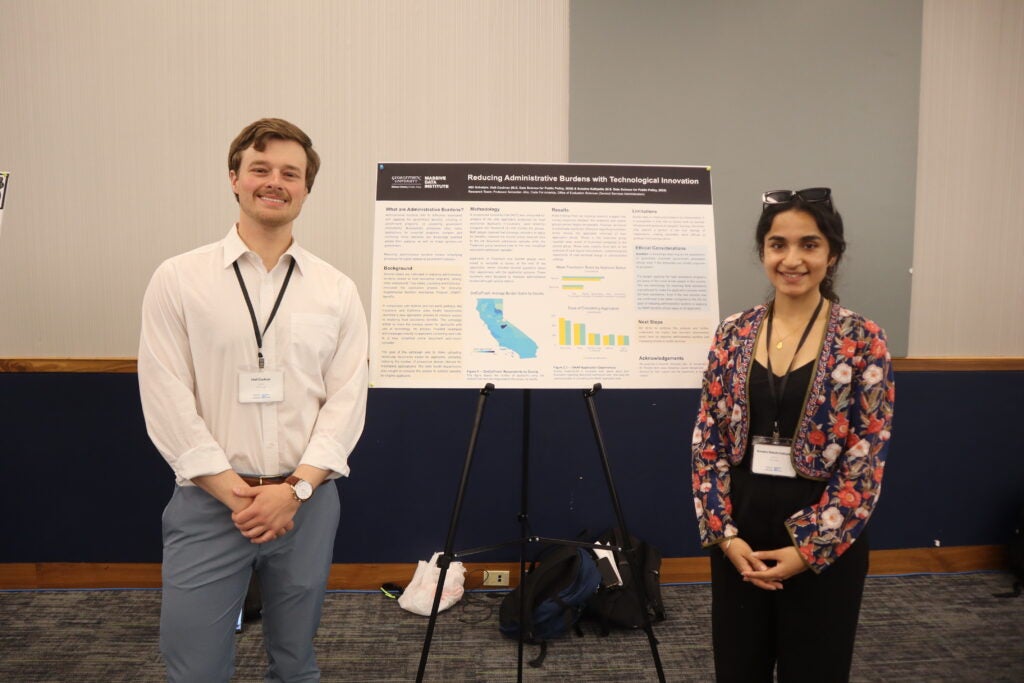
Holt Cochran (MS-DSPP ’25) and Sunaina Kathpalia (MS-DSPP ’25): Reducing Administrative Burdens with Technological Innovation.
Cochran and Kathpalia worked with Professor Sebastian Jilke, Dr. Donald Moynihan, Dr. Pamela Herd, Jason Goodman, and Lauren Spiegel in partnership with Code for America on research into various ways to reduce administrative burdens with technological innovation. Kathpalia explains that their research “looked at whether civic interventions can help reduce these burdens in the context of food assistance programs.” Specifically, Cochran explained that the team looked at the new application processes of Louisiana and California and compared how “attitudes change between people who used the old process and the new process.”
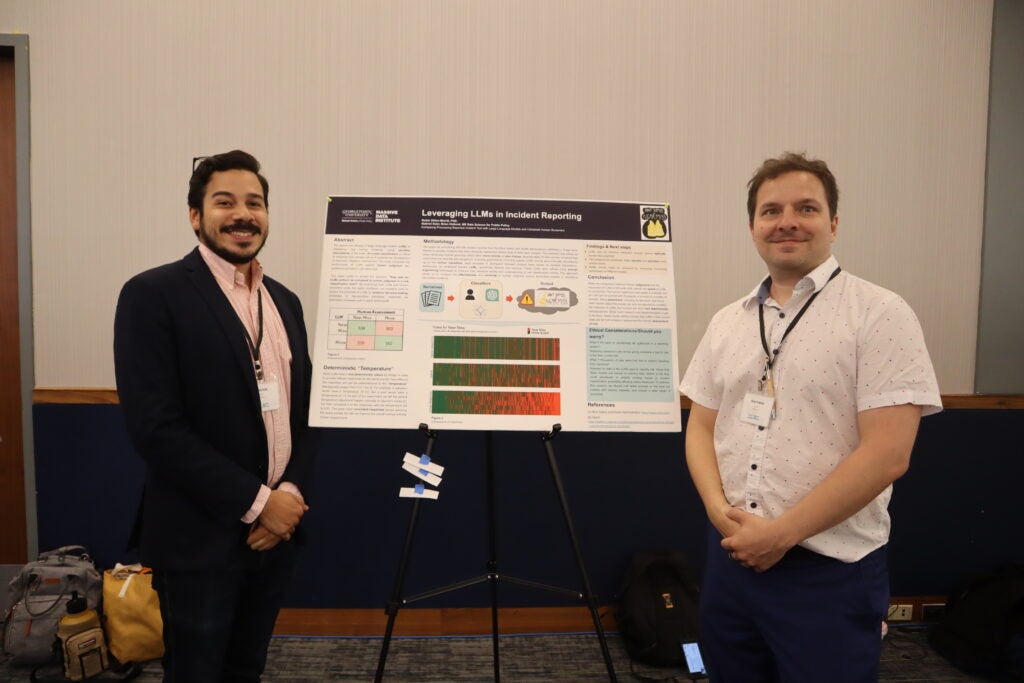
Brian Holland (MS-DSPP ’24) and Gabriel Soto (MS-DSPP ’25): Leveraging LLMs in Incident Reporting.
Holland and Soto worked with Professor Robin Dillon-Merril to research the efficacy of large language models (LLMs) in incident reporting for the mining industry. Comparing the risk assessment capabilities of LLMs with that of humans, Holland explains, “There are many ways that humans are better at assessing risk than LLMs but one area where we found that LLMs crush humans is in speed.”

Amanda Hao (BS-STIA ’26): Compiling Data Sources to Inform Head Start Programs.
Hao worked with Professors Amy O’Hara and Gabriel Taylor to continue her project compiling data sources that allow the National Head Start Association to better understand the reach of their program. Hao explains that her project “explored additional sources to create a streamlined interactive dashboard that fluently displays county and program data,” allowing program managers to compare program performance across the nation and identify areas for improvement.

Xinyu Li (MS-DSAN ’24): Measuring French Racism and Misrepresentation on Social Media to Better Understand Online Perception.
Li worked with faculty advisors Lisa Singh, Andrew Sobanet, and Rokhaya Diallo on a project that aimed to measure French racism and misrepresentation on social media. They sourced posts from X (formerly known as Twitter) and Instagram and categorized the posts by bias to gain insights for potential future data models to measure levels of misrepresentation on social media.
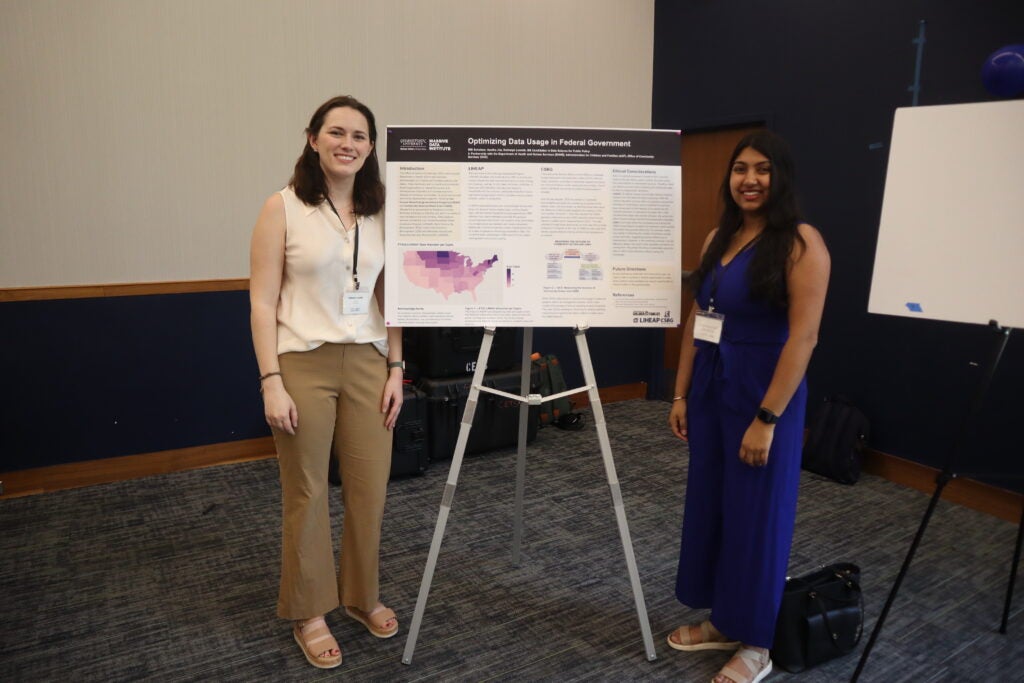
Aastha Jha (MS-DSPP ’25) and Katharyn Loweth (MS-DSPP ’25): Optimizing Data Usage in Federal Government.
Jha and Loweth worked with Professor Michael Bailey in partnership with the Department of Health and Human Services, the Administration for Children and Families, and the Office of Community Services (OCS) on a project that aimed to optimize data usage in the Low Income Home Energy Assistance Program and the Community Services Block Grant. Both these programs are initiatives by the OCS to allocate funds to states, territories, and tribes to improve the lives of low-income families. This project aimed to use this data to make these programs more equitable.
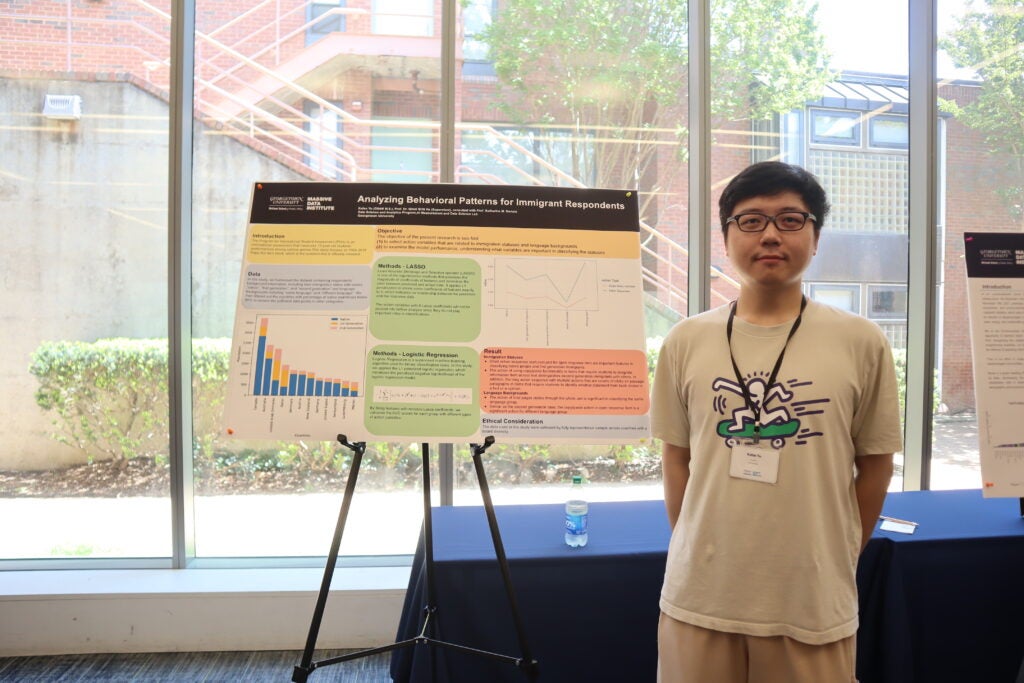
Kefan Yu (MS-DSAN ’24): Analyzing Behavioral Patterns for Immigrant Respondents.
Yu worked with professors Qiwei Britt He and Katharine Donato on a study that used data from the Program for International Student Assessment–a program that measures 15-year-old students’ performances on different tests–to observe the differences in scores among native, first generation, and second generation students. Yu explained that they were “interested in the actions or action sequences that distinguish people with native status from those with first or second generation status,” looking to find trends among assessment participants.

Madhvi Malhotra (MS-DSPP ’24): Federal Spending: Bipartisan Infrastructure Law.
Malhotra worked with Professor Michael Bailey on an Environmental Impact Data Collaborative (EIDC) project. Malhotra explained that this project, titled “Federal Spending: Bipartisan Law”, used data from where? to develop a dashboard that “aimed to track investments” and funding within federal environmental sustainability programs in different regions and communities across the U.S. that are impacted by the Federal Bipartisan Infrastructure Law.

Roy Hwang (BS-Computer Science ’25) and Julia Nonnenkamp (BS-Computer Science & Mathematics ’24): Auditing Probabilistic Genotyping Software Used in Forensic DNA Analysis.
Hwang and Nonnenkamp continued their work with Elissa Redmiles, Lisa Singh, Ionnis Ziogas, Lucy Qin, Christian Schoebert, Yanchen Wang, Stevie Glaberson, and Emily Tucker on a project that investigated the probabilistic genotyping software (PGS) technology used for forensic genetic data analysis. Hwang explained that “most PGS that law enforcement use are commercial and closed-source. The National Institute of Standards and Technology has called for more robust independent testing of this software because they are often intransparent and they have a huge impact on the justice system.” This project used technical analysis to identify possible risks of PGS and the algorithms used by this software.
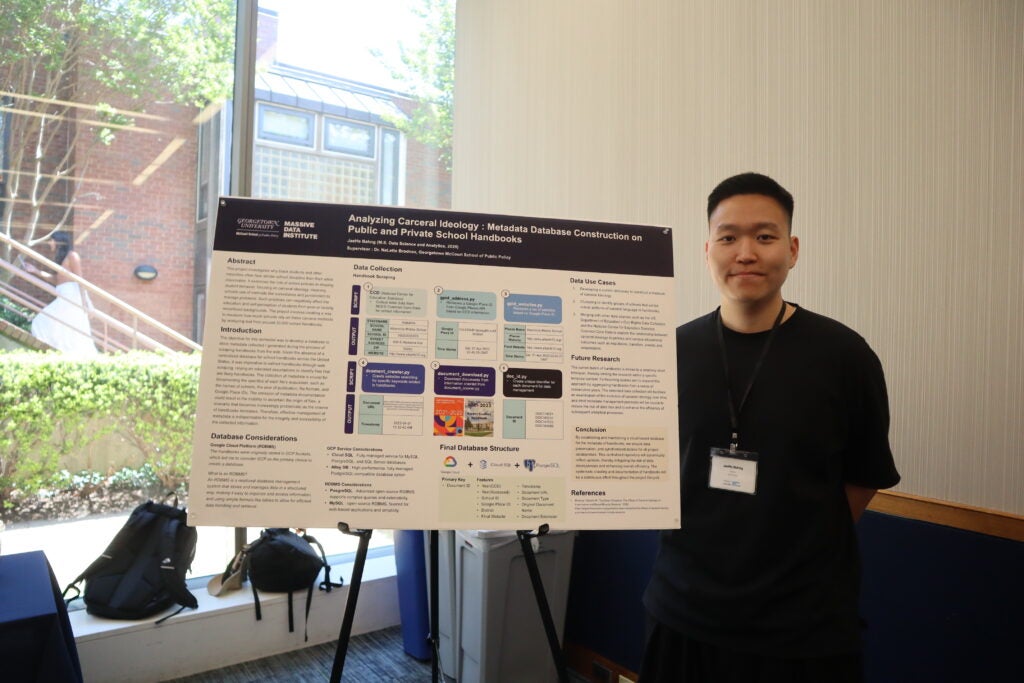
JaeHo Bahng (MS-DSAN ’25): Analyzing Carceral Ideology: Metadata Database Construction on Public and Private School Handbooks.
Bahng worked with Professor NaLette Brodnax to construct a cloud-based database to measure how much schools rely on strict discipline and punishment, and how these policies disproportionately affect minority students. Bahng explained that the aim of this project was to “create a degree of measurement for carceral ideology within the policy of school handbooks and link it to the outcomes of students with minoritized backgrounds.”
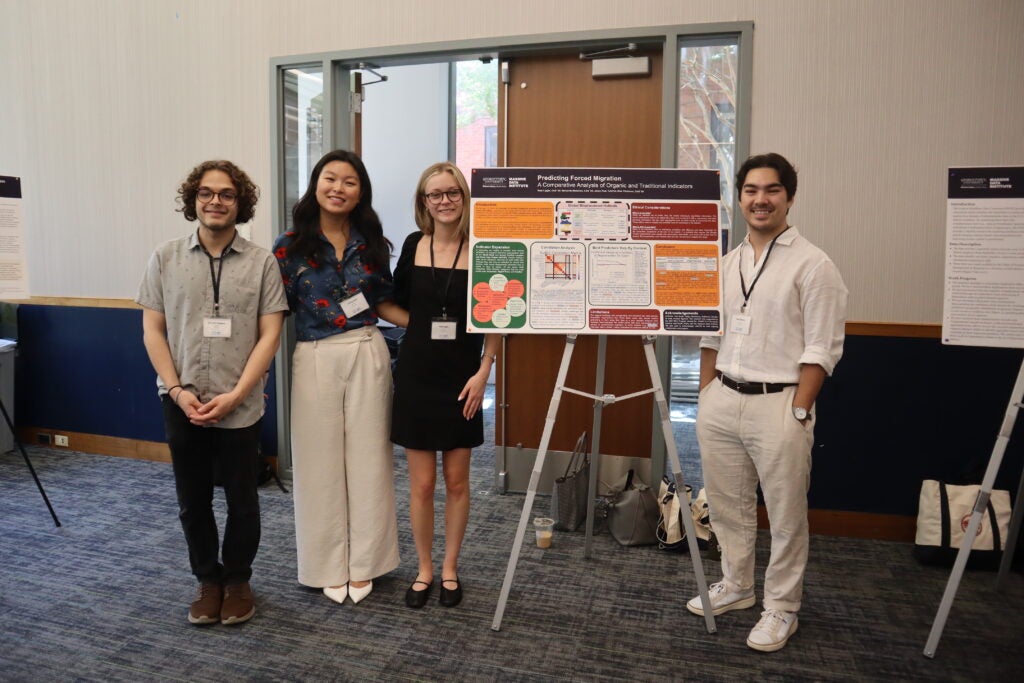
Kate Liggio (BS-Computer Science ’24), Bernardo Madeiros (BS-Computer Science ’24), Jenny Park (BS-Computer Science & Justice and Peace Studies ’24), and Rich Pihlstrom (BS-Computer Science ’24 and MS-DSAN ’25): Predicting Forced Migration, A Comparative Analysis of Organic and Traditional Indicators.
Liggio, Madeiros, Park, and Pihlstrom worked with Lisa Singh, Helge Marahrens, Katherine Donato, Ali Arab, and Ameeta Agrawal on a project aimed at predicting forced migration. Using correlation analysis, the researchers looked at various indicators of migration such as stock data, Google trends, and violent event counts, to try to better predict and map displacement patterns. Pihlstrom explained that “going forward, [the team] wants to continue expanding and diversifying our list of indicators to not only better predict displacement patterns but also to ensure adequate representation of affected populations in our data.”
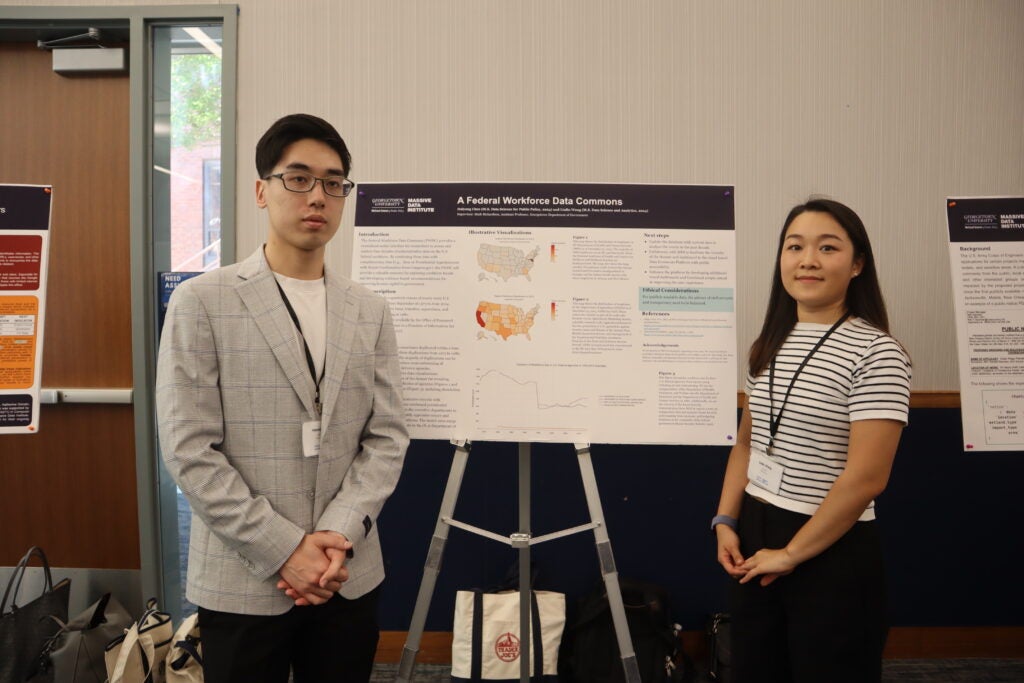
Haiyang Chen (MS-DSPP ’24) and Linlin Wang (MS-DSAN ’24): A Federal Workforce Data Commons.
Chen and Wang worked with Professor Mark Richardson to combine administrative data from the Federal Workforce Data Commons with complementary data to demonstrate workforce trends and shape recommendations for improving human capital in government. Wang explained that their work “provides a centralized online interface for researchers to get access to and analyze this data,” in the hopes of making it more accessible for users.
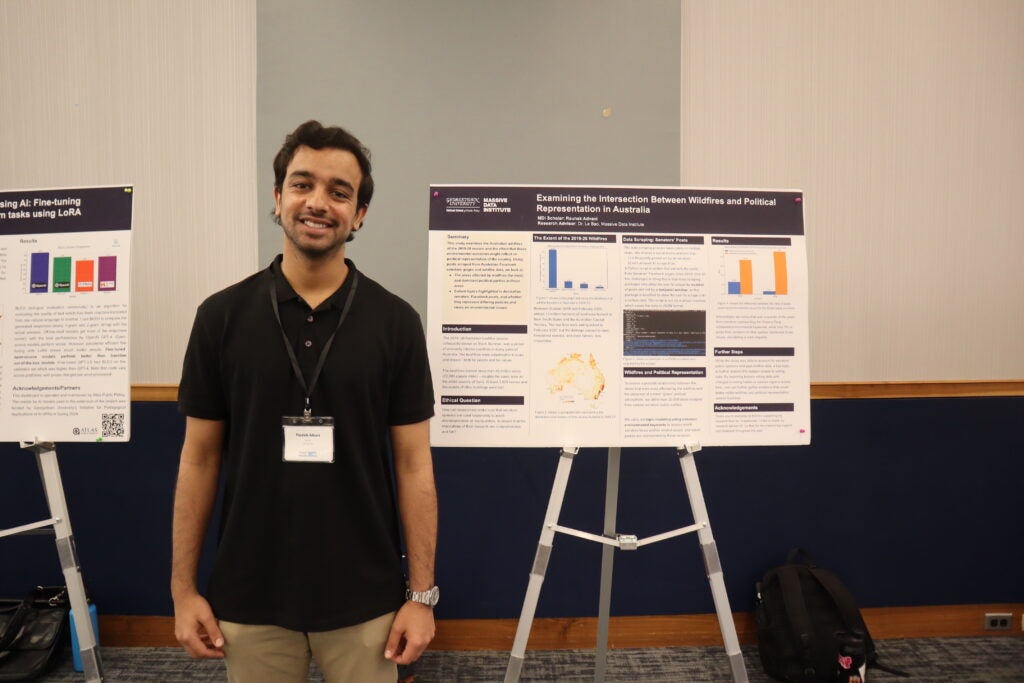
Raunak Advani (MS-DSAN ’24): Examining the Intersection Between Wildfires and Political Representation in Australia
Advani worked with faculty advisor and MDI Fellow Le Bao to examine the relationship between wildfires and political representation in Australia. Advani explained that they “built a webscaper to scrape senators’ posts from Facebook,” and used that data to look at the dominant political parties in regions of Australia that were most affected by wildfires and the amount of environmental discourse in social media posts by senators of those regions. In the future, the team hopes to explore voting data to gain more insights into the relationship between political representation and Australian wildfires.
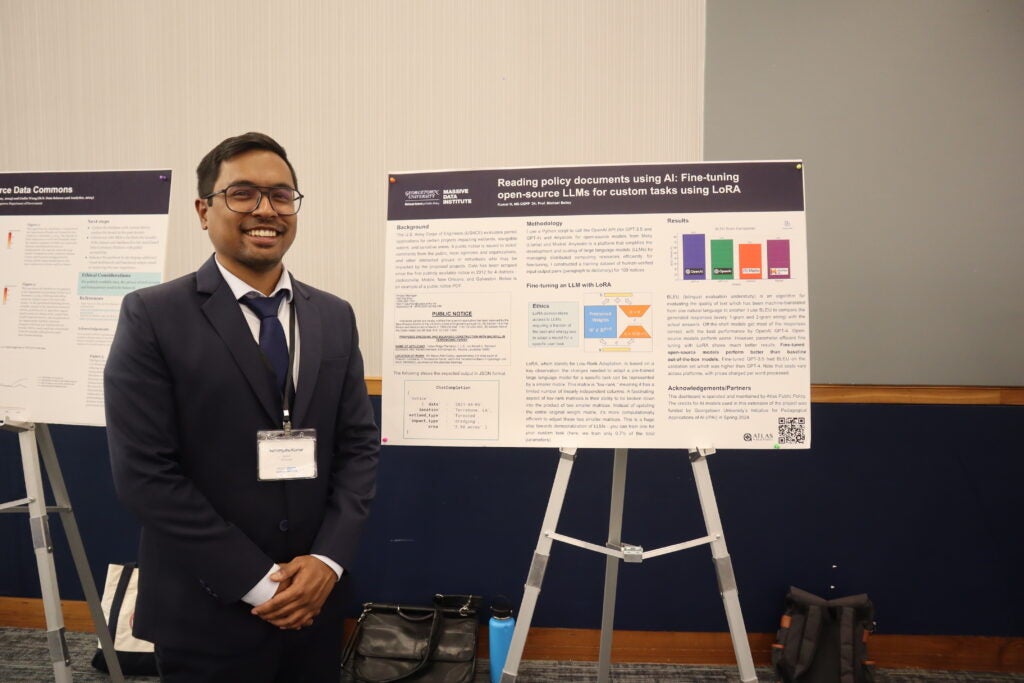
Himangshu Kumar (MS-DSPP ’24): Reading Policy Documents using AI: Fine-tuning Open-Source LLMs for Custom Tasks using LoRA.
Kumar worked with Professor Michael Bailey to continue a project using OpenAI to process public notices uploaded by the U.S. Army Corps of Engineers and extract data on wetland damages. Kumar explained that, when comparing OpenAI to open-source language models, “results showed that you can actually fine-tune open source language models for a few hundred dollars, and [the results] will actually perform equally to GPT-4, or even better in some cases.”
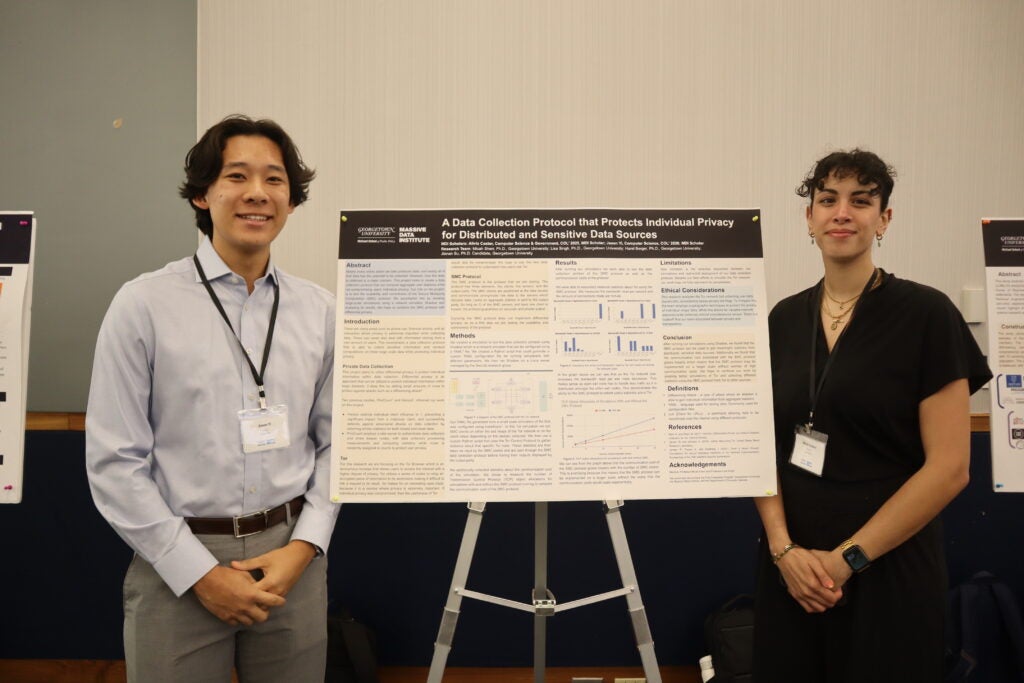
Alivia Castor (BS-Computer Science & Government ’25) and Jason Yi (BS-Computer Science ’26): A Data Collection Protocol that Protects Individual Privacy for Distributed and Sensitive Data Sources.
Castor and Yi worked with advisors Micah Sherr, Lisa Singh, Harel Berger, and Jianan Su on a project seeking to find ways to collect data without compromising the privacy of users. For this project, they tested the accuracy of the Secure Multiparty Computation (SMC) protocol, a system used for data collection. Castor explains that “the SMC protocol shows a lot of promise for collecting statistics securely and has given us a framework to collect information on how data is truly used, which will help us with better regulations.”

Zhiqiang Ji (MS-DSPP ’24): Harnessing AI to Map Political Ideologies: Challenges and Strategies for Cost-Effective Deployment.
Ji worked with Professor Michael Bailey on a project using Large Language Models to analyze ideologies of the U.S. House of Representatives using their statements. Using OpenAI, this research highlighted the potential for Large Language Models to assist with social research. While this project demonstrated some strengths of Large Language Models, Ji explained that “for future research, we are going to extend the analysis to other languages and other political contexts, and try more open-source models.”
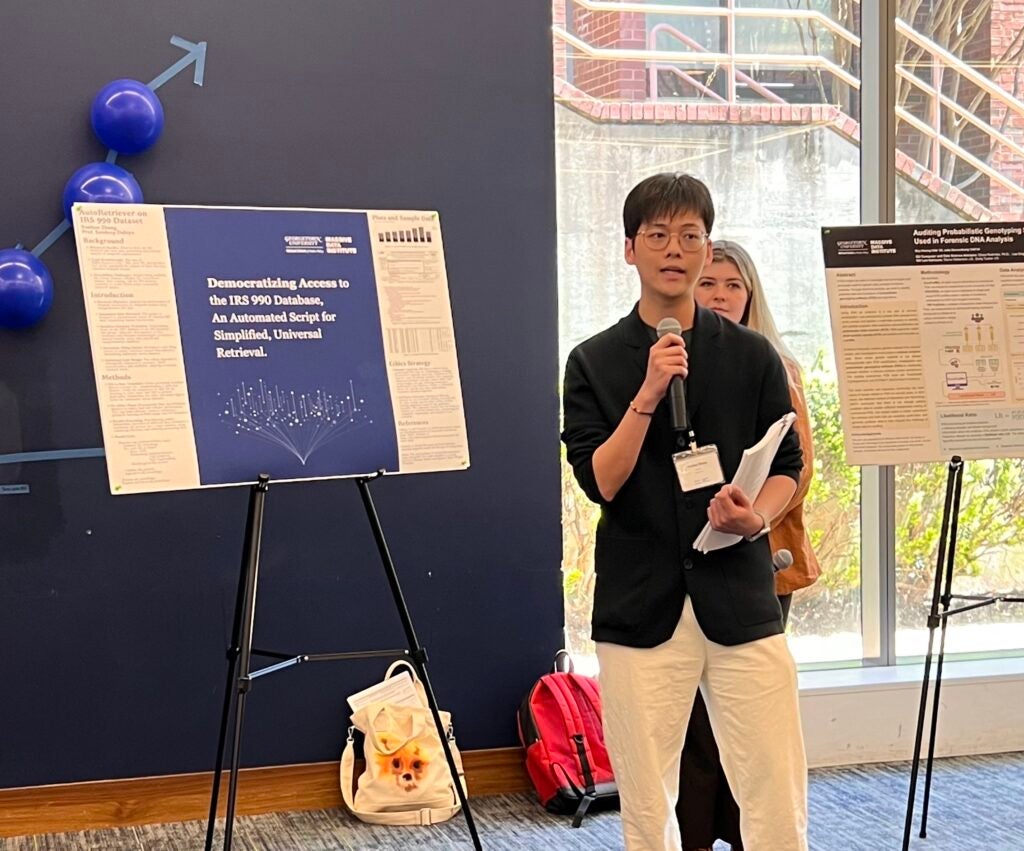
Yunhan Zhang, (MS-DSAN ’24): Democratizing Access to the IRS 990 Database: An Automated Script for Simplified, Universal Retrieval.
Zhang worked with Professor Sandeep Dahiya on a project analyzing the performance of various nonprofits’ financial investments using IRS data. Prior to 2015, the IRS withheld 990 form data, which prevented analysis of these nonprofit organizations. Now that this data is available, this project used automated data retrieval and database transition to map the performance of different investments. Check out the poster here.