Announcing Fall 2024 Research at MDI
The Massive Data Institute at the McCourt School of Public Policy welcomed the Fall 2024 Cohort of student researchers participating in the MDI Scholars Program, the REU program, the Sonneborn program, and independent research endeavors. Representing four colleges across Georgetown University, including the McCourt School of Public Policy, Walsh School of Foreign Service, Graduate School of Arts & Sciences, and the College, 42 Georgetown undergraduate and graduate students will be conducting research alongside Georgetown Faculty.
This semester, student researchers are working on 25 research projects on topics ranging from AI generated art, healthcare, to election dynamics. While some students are participating in the MDI Scholars Program, we also have students working on other research projects with faculty affiliated with MDI.
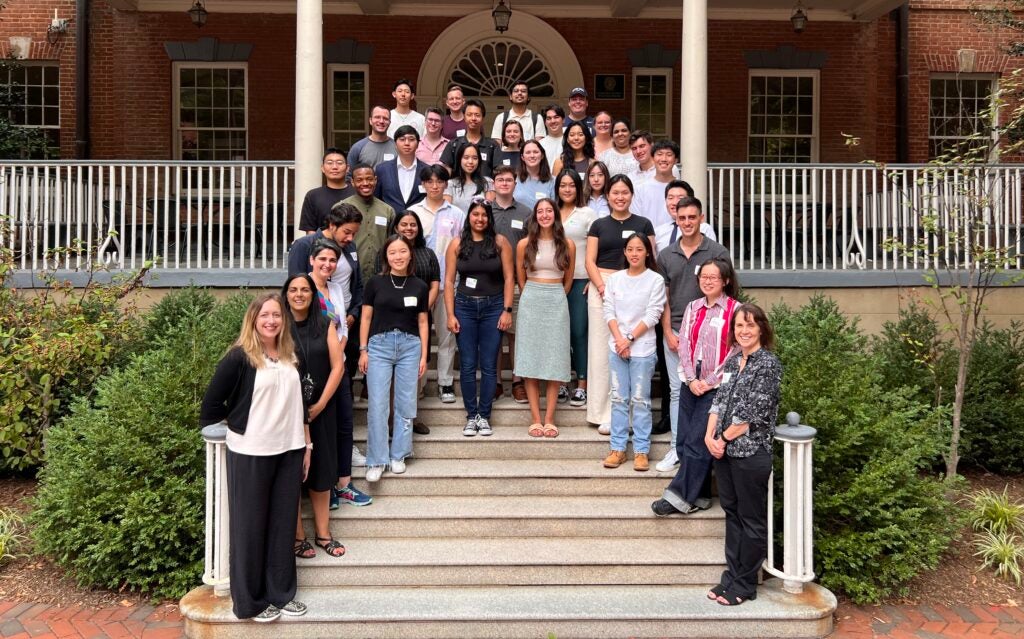
Fall 2024 MDI Student Researchers and Advisors.
Fall 2024 Research Teams
(listed in alphabetical order of advisor)
Characteristics of Medicaid Managed Care Organizations (MMCOs) and their relationship with state-level Medicaid policies and the populations they serve
Advisor: Maria Alva, Assistant Professor
Description: Most states now enroll Medicaid beneficiaries in managed care organizations (MMCOs). Yet, while managed care has become the dominant system for care delivery for this population, we know very little about these organizations’ characteristics, how their presence correlates with state-level Medicaid policies (e.g., capitation rates, mandatory enrollment, patient randomization, and types of covered benefits, including prescriptions), and how plan characteristics (e.g., for-profit/non-for-profit, longevity, states served, market penetration, revenues, and quality of care metrics) correlate with the characteristics of the population they serve (e.g., age, race, and comorbidities).
Student Researcher: Shun Liu ’25, Data Science for Public Policy
Income and Leisure shocks for Patients with chronic conditions and their caregivers
Advisor: Maria Alva, Assistant Professor
Description: This study seeks to answer three questions:
What is the impact of chronic conditions on patients’ and caregivers’ labor-market outcomes?
How do demographic characteristics and socio-economic status moderate the impacts of chronic conditions on patients and caregivers?
How do the burden-specific conditions compare to one another?
Student Researcher: Yixin Luo ’25, Data Science for Public Policy
Data Science for Policy at HHS
Advisor: Michael Bailey, Walsh Professor in the Department of Government and McCourt School of Public Policy
Description: MDI has built a relationship with HHS in which we send students to work with them on data science projects. To date, the projects have been related to the environment, with a focus on the Low-Income Home Energy Assistance Program (LIHEAP). We have automated the calculation of their complex formula for distributing funds to states. We have also worked on identifying regions particularly vulnerable to heat-stress. Aastha will continue on the automation project and we expect her to also to add new projects.
Student Researcher: Aastha Jha ’25, Data Science for Public Policy
Text to Ideology
Advisor: Michael Bailey, Walsh Professor in the Department of Government and McCourt School of Public Policy
Description: Political actors express their views in many ways; understanding these views is important to understanding who gets elected, who raises money and who is extreme. Converting text into measurable ideology is not simple however, as the relationship between ideology and rhetoric is more subtle than the relationship between legislative votes and ideology. We are working to develop models that allow us to measure ideology of political actors based on text on their campaign websites and on social media. Importantly, this allows us to measure virtually every candidate for U.S. Congress, thereby making it possible for us to evaluate the connection between ideology, election outcomes and electoral institutions.
Student Researcher: Quan Yuan ’25, Master of Science in Data Science and Analytics
Investigating the Risk of Identification of Healthcare-Seeking Behavior from Aggregated Mobility Data: Balancing Public Health Needs and Privacy Issues
Advisor(s): Shweta Bansal, Professor of Biology and Giulia Pullano, Postdoctoral Associate
Description: This project explores how to balance the use of high-resolution mobility data for public health purposes while addressing privacy concerns related to the identification of sensitive healthcare-seeking behavior. To do that, we will apply privacy-preserving techniques to mobility data, and we will assess the effectiveness of the private versus no-private mobility data in generating public health insights and guiding interventions.
Student Researchers: Yundi (Wendy) Shi
AI-Generated Images and Identifiability
Advisors: Sarah Adel Bargal, Assistant Professor of Computer Science and Provost’s Distinguished Faculty Fellow; Elissa Redmiles, Clare Luce Boothe Assistant Professor in the Department of Computer Science; Rupayan Mallick, Postdoctoral Fellow at the Massive Data Institute and the Department of Computer Science
Description: Technological advancements in Artificial Intelligence (AI) have enabled the creation of synthetic image, audio, and video representations of individuals. It is impossible to establish theoretical guarantees that these models will not produce content depicting a person without their consent. Thus, our project aims to assess the risk of generative models being used to produce non-consensual images that can be shared publicly and cause harm. Specifically, we evaluate the propensity of AI models to generate faces of a specific identity with the goal of developing technical mechanisms to reduce non-consensual generation of such images.
Student Researcher: Jason Yi ’26, Bachelor of Science in Computer Science
AI-based Story Generation
Advisors: Sarah Adel Bargal, Assistant Professor of Computer Science and Provost’s Distinguished Faculty Fellow; Rupayan Mallick, Postdoctoral Fellow at the Massive Data Institute and the Department of Computer Science
Description: Diffusion-based models are increasingly becoming state-of-the-art in various generative AI tasks. In this project, we aim to push state-of-the-art for the story generation task.
Student Researchers: Xinhe (Maggie) Shen ’26, Bachelor of Science in Computer Science and Mathematics; Sibo Dong, PhD student in Computer Science Department
Identification of Sentinel Near Misses for Predictive Safety: Leveraging AI for Incident Identification and Risk Forecasting
Advisor: Robin Dillon-Merrill, Professor and the Operations and Analytics Area Chair in the McDonough School of Business
Description:Using a dataset of U.S. coal mining incident reports and human coders, we are identifying sentinel near misses using the human applied labels to a fraction of the reports to train a ML model to classify the remaining reports.
Student Researcher: Gabriel Soto ’25, Data Science for Public Policy
The Role of Near Misses in Decision Making for Autonomous Vehicles
Advisor: Robin Dillon-Merrill, Professor and the Operations and Analytics Area Chair in the McDonough School of Business
Description: We are exploring data collected for an autonomous vessel operated by researchers in the Fjords of Trondheim Norway to understand how near misses can influence the vessel’s decision making.
Student Researcher: V. Sahasra Bandaru ’25, Master of Science in Computer Science
Advancing Sequence Clustering with Image Processing in Educational Assessments
Advisor: Qiwei Britt He, Associate Professor in Data Science and Analytics Program
Description: Scenario-based interactive tasks are increasingly used in educational assessments nowadays. This innovative design records all human-computer interactions in log files, providing detailed information to better understand students’ behaviors and problem-solving strategies. In this project, we will utilize process data from the Program for the International Assessment of Adult Competencies (PIAAC) to develop a new method for sequence clustering using image processing. By analyzing pixels across each pair of images from students’ process data, we aim to significantly improve the accuracy of sequence similarity computations and better cluster similar patterns together.
Student Researchers: Binhui Chen ’25, Master of Science in Data Science and Analytics; Sibo Dong, PhD student in Computer Science Department
Addressing Inequality Through Supreme Court Cases: Amicus Briefs from Asian, Latino, and Black Advocacy Groups (1970-2021)
Advisor: Helge Marahrens, Postdoctoral Fellow at the Massive Data Institute; Muna Adem (University of Maryland); Dina Okamoto (Indiana University)
Description: This project aims to understand cooperation and conflict between ethnoracial advocacy groups from 1970 to 2021. We analyze amicus briefs, legal documents that allow organizations to offer additional perspectives, expertise, or information that could help the Supreme Court in its decision-making process. We use network analysis to examine co-signatures and text analysis to understand which types of cases (e.g., topics) facilitate cooperation or conflict.
Student Researcher: Zining (Cathy) Wang ’25, Master of Science in Data Science and Analytics
Restoring the Health of the Election Information Ecosystem: The Election Officials’ Communications Tracker
Advisor: Thessalia Merivaki, Associate Teaching Professor, McCourt School of Public Policy, and Associate Research Professor, Massive Data Institute
Description: This project aims to identify communication strategies election officials use online to inform the public about how to participate in elections, and build trust in the electoral process. Using manual quantitative coding methods and automated encoding procedures using LLMs, we monitor and label communications shared by state and local election officials on social media.
Student Researchers: Jorge Bris Moreno ’25, Master of Science in Data Science and Analytics; Priyasha Chakravarti, Undergraduate Student; Aditya Vishahan, Undergraduate Student
Managing Brand Crisis and Customer Relationships on Social Media: Insights from Twitter in the Airline Industry
Advisor: Emisa Nategh, Assistant Teaching Professor of Operations and Analytics at McDonough School of Business
Description: This study explores how customer feedback on social media impacts brand perception, revenue, quality outcomes, and operational decisions in the airline industry. It demonstrates that firms use the emotional content of this feedback in their management strategies. Additionally, we examine how the political affiliations of airlines influence their customer service.
Student Researcher: Zoucheng Hong ’25, Master of Science in Mathematics and Statistics
Guiding Educators on Sharing and Protecting Student Data through Privacy Enhancing Technologies
Advisors: Amy O’Hara, Research Professor at MDI and Executive Director of the Georgetown Federal Statistical Research Data Center at the McCourt School for Public Policy; Stephanie Straus, Policy Fellow at MDI
Description: Privacy Enhancing Technologies (PETs) allow for increased data sharing and access while simultaneously preserving the utility and privacy of that data. MDI is assisting state and local education agencies in implementing PET pilots that fill a data gap in their student longitudinal data systems. In addition to these pilots, MDI has created a website of resources for education data owners that includes a bibliography on existing PETs, real-world examples, and a PET 101 training series. https://mdi.georgetown.edu/privacy-enhancing-technologies
Student Researchers: Victor Chen ’27, Bachelor of Science in Computer Science; Camille Deschapelles ’26, Undergraduate student; Justin Liu ’26, Master of Public Policy
Developing a Secure Query System to Measure Earnings and Employment Outcomes
Advisor: Amy O’Hara, Research Professor at MDI and Executive Director of the Georgetown Federal Statistical Research Data Center at the McCourt School for Public Policy
Description: The IRS Secure Query System (SQS) will link state and local agency data to IRS records to generate aggregate statistics. Currently in development, SQS features administrative processes to determine eligibility and enroll clients, a tool to validate data on client side before files are shared with IRS, an automated matching process within IRS (by SOI employees), tabulation of pre-defined statistics, and an automated disclosure avoidance review. Students are assisting with coding, testing, and research to improve matching and disclosure avoidance methods.
Student Researcher: Kangheng Liu ’25, Master of Science in Data Science and Analytics
Analyzing Juvenile Court Data
Advisors: Amy O’Hara, Research Professor at MDI and Executive Director of the Georgetown Federal Statistical Research Data Center at the McCourt School for Public Policy; James Carey, Legal Fellow at MDI
Description: State court data on juvenile records are collected for administrative, not secondary, use. This project develops methods to assess data quality and completeness, and to produce actionable reports that maintain privacy over sensitive case details about youth and judicial staff alike.
Student Researcher: Sheeba Moghal ’25, Master of Science in Data Science and Analytics
Genetic Data, Algorithmic Outputs, and Explainability
Advisors: Elissa Redmiles, Clare Luce Boothe Assistant Professor in the Department of Computer Science; Lucy Qin, Postdoctoral Fellow at the Massive Data Institute; Lisa Singh, MDI Director, Chair of the Department of Computer Science, Professor in the Department of Computer Science and McCourt School of Public Policy, Sonneborn Chair for Interdisciplinary Collaboration
Description: This project further examines how the outputs of probabilistic genotyping software (PGS) are interpreted in court processes. When DNA evidence is found at a crime scene, it is analyzed using PGS systems that then produce statistics to convey the likelihood around the similarity between a suspect’s DNA and DNA evidence. Our project aims to understand how these statistical results are explained to laypeople (as a stand-in for jurors) and others that have decision-making power in court processes. Specifically, we will conduct a study to evaluate people’s understanding of these statistical results (based on different explanations) and how this might influence further decision-making.
Student Researchers: Jeffrey Gao ’25, Bachelor of Science in International Political Economy; Miranda Xiong ’24, Bachelor of Arts in History and Classics
Experiential Research into Perceptions of Ownership over AI Generated Art
Advisors: Toni-Lee Sangastiano, Digital Media Specialist and Associate Professor of the Practice in the Department of Art & Art History, and Medical Humanities Core Faculty; Kristelia García, Anne Fleming Research Professor of Law, Institute for Technology, Law & Policy; Elissa Redmiles, Clare Luce Boothe Assistant Professor in the Department of Computer
Description: How do lay people perceive AI-generated artistic outputs with regards to authorship and the protection of AI-generated art? In order to collect perception data that can inform future legislation regarding public and stakeholder opinion. Specifically, we plan to conduct a series of experiential research experiments in the form of juried art competitions with at least four groups of stakeholders in order to gain a robust socio-technical understanding of authorship, incentives, and values.
Student Researcher: Hexuan Wang ’27, Bachelor of Art (undeclared)
Computational analysis of negative conjunction and disjunction in the US Code
Advisor(s): Nathan Schneider, Associate Professor of Linguistics & Computer Science; Brandon Waldon, Postdoctoral Fellow at the Massive Data Institute and in the Departments of Linguistics & Computer Science
Description: In US law, the framework known as legal textualism is associated with several “canons of construction” which codify heuristics for disambiguating difficult statutes. One such canon, the conjunctive/disjunctive canon, resolves ambiguities which arise when negation words (e.g., “not”) appear with connectives (e.g., “and” and “or”), as in “don’t text and drive” (~ don’t do both simultaneously) or “don’t drink and smoke” (~ don’t drink *and* don’t smoke). Using techniques from Natural Language Processing (NLP), we empirically test the reliability of the conjunctive/disjunctive canon by analyzing how such ambiguities are resolved in the US Code. This project demonstrates how NLP can help lawyers, judges, and the public navigate difficult problems of legal text analysis.
Student Researcher: Micaela Wells ’26, Bachelor of Arts in Linguistics
Data Collection from Distributed Sensitive Sources
Advisors: Micah Sherr, Callahan Family Professor of Computer Science in the Department of Computer Science; Lisa Singh, MDI Director, Chair of the Department of Computer Science, Professor in the Department of Computer Science and McCourt School of Public Policy, Sonneborn Chair for Interdisciplinary Collaboration
Description: This project develops techniques for securely and privately aggregating potentially sensitive data from a diverse and distributed set of data owners. The project supports data-driven decision-making by enabling data sharing that would otherwise not be possible.
Student Researchers: Xinhe (Maggie) Shen ’26, Bachelor of Science in Computer Science and Mathematics; Joshua Wiesenfeld ’25, Bachelor of Science in Computer Science
2024 Election Misinformation
Advisor: Lisa Singh, MDI Director, Chair of the Department of Computer Science, Professor in the Department of Computer Science and McCourt School of Public Policy, Sonneborn Chair for Interdisciplinary Collaboration
Description: As people increasingly consume the news through social media this results in widespread diffusion of misinformation. This project focuses on emerging misinformation detection using a combination of candidate conversation, survey responses, newspaper articles, social media posts, and search trends in the summer leading up to the 2024 Presidential elections.
Student Researchers: Aiden Ehrenreich ’27, Bachelor of Science in Computer Science; Amy Li ’26, Bachelor of Science in Business and Global Affairs/Statistics and French; Ann Lian ’25, Data Science for Public Policy; Rich Pihlstrom ’25, Master of Science in Data Science and Analytics
Election, Misinformation & Immigration and the Americas
Advisors: Lisa Singh, MDI Director, Chair of the Department of Computer Science, Professor in the Department of Computer Science and McCourt School of Public Policy, Sonneborn Chair for Interdisciplinary Collaboration; Katherine Donato, Donald G. Herzberg Professor of International Migration, Sonneborn Chair for Interdisciplinary Collaboration; Ali Arab, Associate Professor in the Department of Mathematics and Statistics, Sonneborn Chair for Interdisciplinary Collaboration
Description: This interdisciplinary forced migration project uses a unique combination of administrative, organic (social media, Google trends, newspapers, etc.), and survey data to improve our understanding of international migration flows from South and Central America to the US and Canadian border. This fall, using different social media platforms, students will work to understand signals in the Americas and the role of misinformation.
Student Researchers: Adrian David Frauca ’27, Bachelor of Science in Computer Science; Katie Merrill ’27, Bachelor of Science in Computer Science; Lauren Stipe ’25, Bachelor of Science in Science, Technology and International Affairs; Mandy Sun ’25, Bachelor of Science in Computer Science; Sergio Rodriguez Cifuentes ’25, Bachelor of Science in International Economics; Sheryn Livingstone ’26, Bachelor of Science in Business and Global Affairs
Humanness
Advisors: Lisa Singh, MDI Director, Chair of the Department of Computer Science, Professor in the Department of Computer Science and McCourt School of Public Policy, Sonneborn Chair for Interdisciplinary Collaboration; Leticia Bode, Professor in the Communication, Culture, and Technology Master’s Program and Research Director of the Knight-Georgetown Institute; Tiago Ventura, Assistant Professor in Computational Social Science at the McCourt School of Public Policy; Sejin Paik, Postdoctoral Fellow at the Massive Data Institute
Description: This research project investigates how well humans can distinguish AI generated posts, from human authors, and the effectiveness of various embeddings and neural network architectures for classifying humanness using social media data from YouTube and X.
Student Researcher: Rebecca Ansell ’25
Predicting Interstate Conflict Using Neural Networks
Advisor: Ioannis Ziogas, Assistant Teaching Professor at the McCourt School of Public Policy and an Assistant Research Professor at MDI
Description: International conflict research has primarily relied on survival models for nearly two decades. This project breaks from that tradition by developing a novel neural network architecture tailored to the unique characteristics and limitations of conflict data. Our approach not only addresses data-driven and model-specific challenges, but also enhances predictive capacity when compared to popular epidemiological deep learning alternatives.
Student Researcher: Billy McGloin ’25, Master of Science in Data Science and Analytics
- Tagged
- MDI Scholars