Comparing State Climate Policy Commitments Over Time
How a combination of domain knowledge and data science can enhance visibility into climate policy challenges
Over the past year and a half, I worked on an MDI research project with my advisor Dr. Parrish Bergquist and our collaborator Dr. Chris Warshaw. Our research objective was to understand the impact of each state’s comprehensive policy regime on its ability to transition to a cleaner energy system. My interest in the project was both academic and personal, having worked in the field of clean energy prior to starting my Master’s at Georgetown in 2020.
In the United States, state governments have been the locus of action for addressing climate change in the face of a federal stalemate. I saw this firsthand in my work at a clean energy trade association, where we worked on passing state legislative packages such as the Virginia Clean Economy Act. But there is no comprehensive index of the stringency of state climate change policies, a domain that is riddled with complexity due to the wide variety of policies that states enact. The lack of a holistic measure of state climate policy regimes has hamstrung efforts to evaluate the effectiveness of states’ efforts to transition to a cleaner energy system.
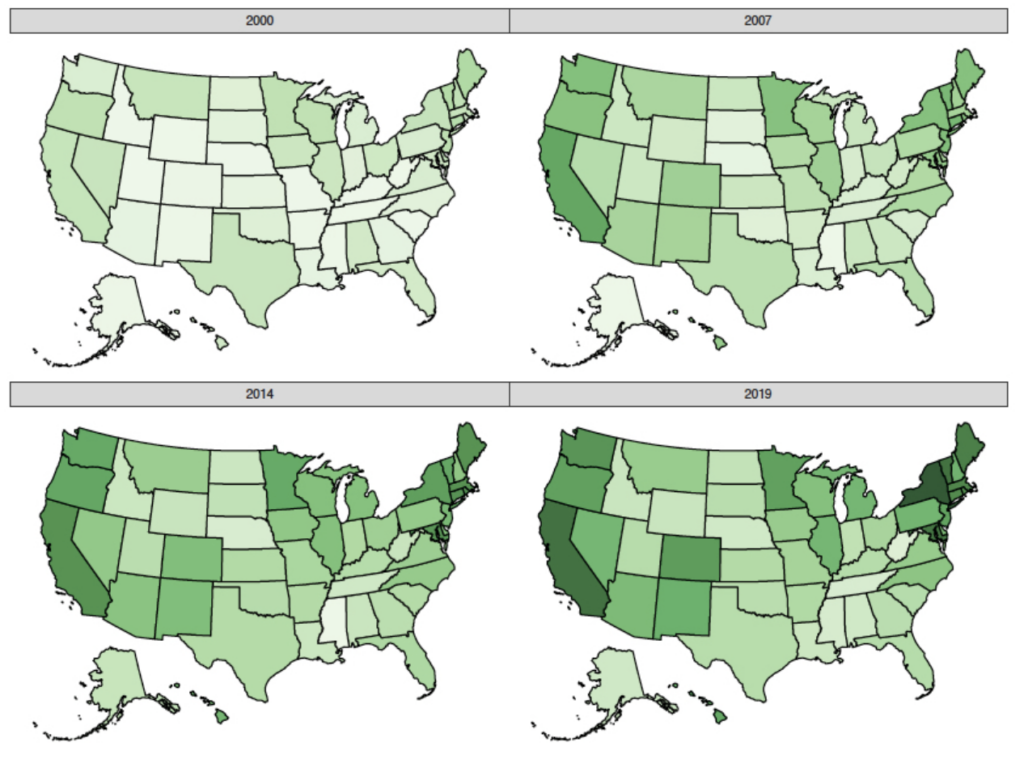
We decided to tackle this by aggregating information from nearly 20 relevant policies to develop a climate policy index to measure each state’s commitment to reducing greenhouse gas emissions between 2000 and 2019. As a researcher on the team, I was responsible for updating, cleaning, and gathering data to serve as inputs to the index. I coded whether each state had enacted each policy in each year. To the extent feasible, I developed an ordinal coding scheme for each policy that reflected variation in legal and regulatory stringency between states (e.g., voluntary vs. mandatory standards). I gathered data from a variety of sources, including scholarship in the field, Correlates of State Policy Project, and various industry and non-profit databases.
This data was used to construct a dynamic item response theory (IRT) model using RStan; the model estimates a measure of climate policy stringency that can be compared across states and over time. This index follows a modeling approach based on Bayesian factor analysis, which processes mixed ordinal and continuous data. We then used regression modeling to examine the impact of climate policy stringency on CO2 (carbon dioxide) emissions, as well as on electricity generation and consumption patterns.
Below, you can see some of our preliminary results. The models show a strong and robust relationship between energy policy and CO2 emissions. A standard-deviation increase in climate policy stringency is associated with a 9.5 percent reduction in annual CO2 emissions from the electricity sector, and a 3.7 percent reduction in CO2 emissions across the economy in a state.
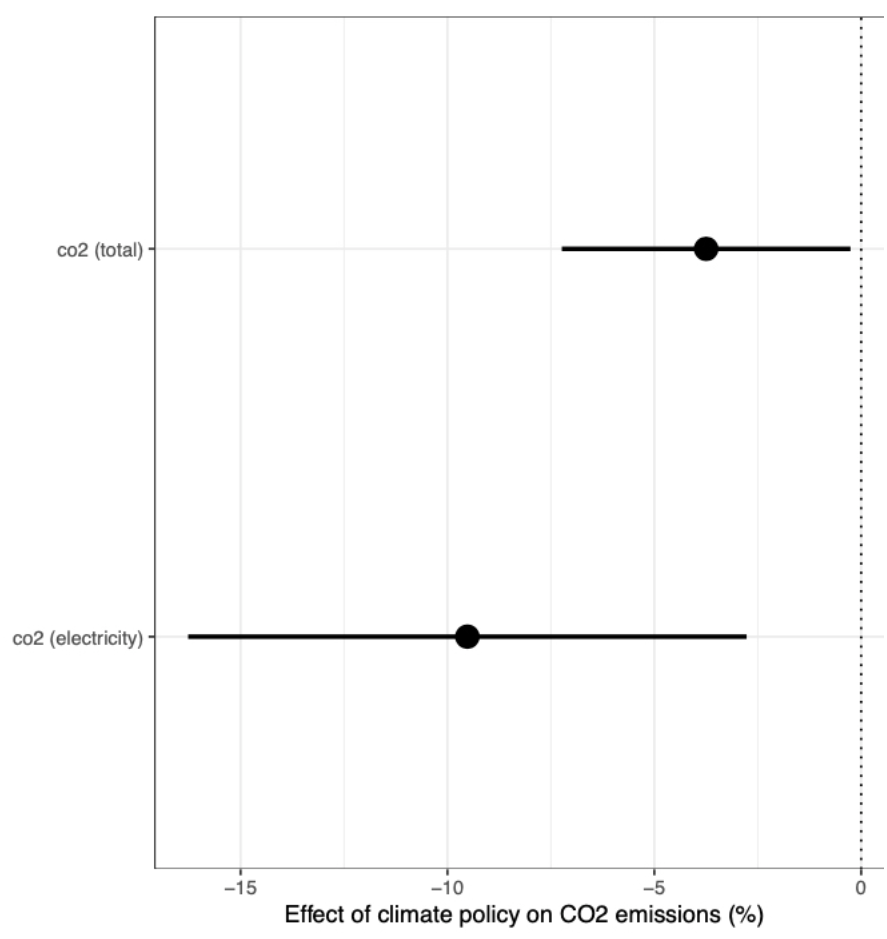
One of the most exciting moments for me was when we validated our resulting index with an industry-standard ranking system – the American Council for Energy Efficiency’s (ACEEE) – annual state scorecard. For multiple years in the time series, we found a 90% correlation between our index and the ACEEE scorecard. This was much higher than I expected given the different inputs to both indices and showed that our index does target an underlying climate policy structure. This was a critical insight as it confirmed that our index can be used as a method of comparison between states.
My time as an MDI scholar, both working for Dr. Bergquist and interacting with scholars working on other research projects, provided me with one key takeaway: the idea of “big data” or “machine learning” can be mystifying to policy researchers and policymakers alike. While progress on the edge of computing is exciting, it is important to identify what the useful applications of these technologies are – and what they are not.
Domain context is invaluable for social science and policy-adjacent research, and it cannot yet be supplanted by machines. For example, in this project, deconflicting the data inputs oftentimes involved going back to original dockets or legislative text manually to find the most appropriate values. This process was informed by my prior understanding of the field.
I will be taking these insights with me into the professional world, along with the recognition that the nexus that I seek between energy policy and data may not be what I expected, and that a human understanding of these systems is critical in continuing research.
Written by Gloria Li, MDI Scholar